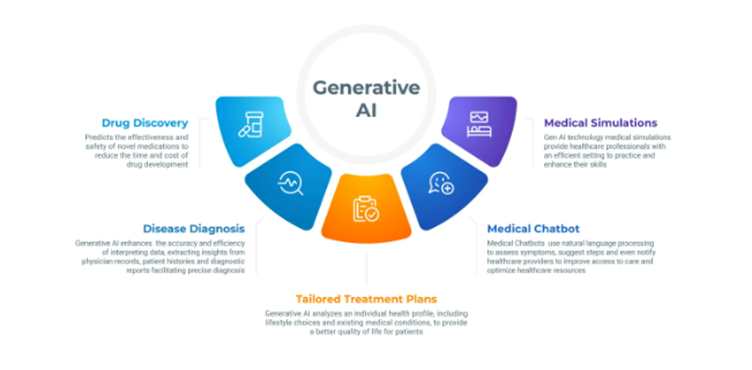
The intersection of Generative AI (GenAI) and bioinformatics is unlocking groundbreaking possibilities in biological research, healthcare, and drug discovery. As artificial intelligence continues to evolve, its ability to generate, simulate, and analyze biological data is reshaping the landscape of life sciences. From designing new proteins to revolutionizing precision medicine, Gen AI and the future of bioinformatics is poised to bring unprecedented advancements.
Generative AI is poised to revolutionize bioinformatics by transforming the way researchers analyze biological data, discover new drugs, and understand complex biological systems through machine learning models. As computational power continues to grow, the integration of AI models in bioinformatics will open up new frontiers in precision medicine, genomic analysis, and synthetic biology. Below are some key areas where generative AI and the future of bioinformatics is expected to make a significant impact.

Understanding Generative AI in Bioinformatics
Generative AI refers to machine learning models that can create new data similar to existing datasets. In bioinformatics, it is used to generate biological sequences, simulate molecular interactions, and predict structural configurations with high precision.
GenAI models like Variational Autoencoders (VAEs), Generative Adversarial Networks (GANs), and Transformer-based architectures (such as AlphaFold and ESMFold) are already demonstrating their potential in areas such as drug design, druf, and genome analysis.
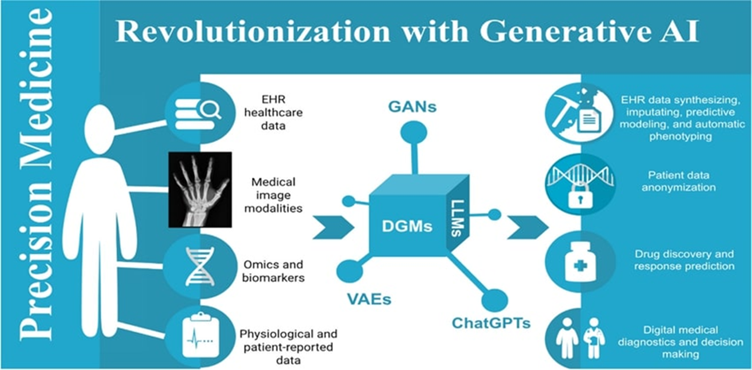
Key Areas Where Generative AI And the Future Of Bioinformatics is Transformed
1. Accelerating Drug Discovery and Development
One of the most promising applications of generative AI in bioinformatics is in drug discovery. AI-powered models can generate novel molecular structures, predict drug-target interactions, and optimize lead compounds, significantly reducing the time and cost associated with traditional drug development. With techniques such as deep learning-based molecular docking and de novo drug design, AI can identify potential therapeutic candidates at an unprecedented speed.
GenAI can significantly speed up this process by:
- Designing novel chemical compounds with desired properties.
- Predicting drug-target interactions and side effects.
- Optimizing molecular structures for enhanced efficiency.
- Reducing the need for extensive lab testing by simulating drug behavior.
Future Potential:
- AI-generated drug candidates could reduce development time from years to months.
- Virtual drug testing using AI could minimize the reliance on animal and human trials, thus revolutionizing generative AI and the future of bioinformatics.
- Personalized drug design based on an individual’s genetic makeup.
2. Genomics and Personalized Medicine
Generative AI can assist in analyzing large-scale genomic datasets to identify mutations, predict disease risks, and tailor personalized treatment plans. Machine learning- driven models can generate synthetic genomic sequences to simulate rare genetic variations, improving the accuracy of predictive models. This advancement will play a crucial role in precision medicine, enabling clinicians to develop targeted therapies based on an individual’s genetic makeup.
GenAI is revolutionizing genome analysis and personalized medicine by:
- Generating synthetic genomic data to enhance research.
- Predicting disease risks based on genetic variations.
- Identifying genetic mutations responsible for inherited diseases.
- Designing personalized treatments based on an individual’s genetic profile.
Future Potential:
- AI-powered genome sequencing could enable real-time disease detection.
- Predictive models could forecast how patients respond to specific treatments.
- Synthetic genomes could help researchers study rare diseases without relying on actual patient data.
3. Protein Structure Prediction and Engineering
The prediction of protein structures is essential for understanding biological functions and designing new proteins for medical and industrial applications. Generative AI models, such as AlphaFold, have already made significant strides in this field. Future advancements will further enhance the accuracy of protein structure prediction, facilitate protein engineering, and enable the development of novel enzymes for biotechnology and therapeutic applications.
Gen AI and the future of bioinformatics can further advance by:
- Designing new proteins with customized functions.
- Predicting the impact of mutations on protein structure.
- Simulating protein-drug interactions for better therapeutic solutions.
Future Potential:
- AI could design synthetic enzymes for industrial and medical applications.
- Protein-based therapeutics could be engineered with higher specificity and fewer side effects.
- AI-driven antibody and vaccine development could accelerate responses to emerging diseases.
4. Enhancing Biomedical Image Analysis
AI-driven image generation and enhancement techniques are transforming biomedical imaging and diagnostics. Generative AI can create high-resolution medical images, detect anomalies in biological tissues, and assist in automated disease diagnosis. These advancements will improve the accuracy and efficiency of medical imaging, aiding radiologists and pathologists in detecting diseases at an early stage.
Medical imaging plays a vital role in diagnostics, and Gen AI and the future of bioinformatics can enhance this by:
- Generating high-resolution medical images from limited data.
- Automating disease detection through image synthesis and enhancement.
- Predicting tumor growth and disease progression.
Future Potential:
- AI-powered radiology could reduce human errors in diagnosis.
- Virtual simulations of disease progression could help in preventive medicine.
- AI-generated medical data could augment clinical training and research.
5. Synthetic Data Generation for Research
One of the challenges in bioinformatics is the limited availability of high-quality biological data. Generative AI can create synthetic datasets that closely resemble real-world biological data, allowing researchers to train machine learning models without privacy concerns. This will accelerate AI research in healthcare while ensuring data security and ethical considerations.
Generative AI can create synthetic but realistic datasets, which can be used for:
- Training AI models without exposing patient data.
- Simulating biological experiments without conducting them physically.
- Augmenting clinical datasets to improve machine learning predictions.
Future Potential:
- AI-generated data could solve data scarcity issues in rare diseases.
- Researchers could test hypotheses faster without real-world limitations.
- Secure AI-generated datasets could enhance collaboration across institutions while maintaining privacy.
6. AI-Powered Evolutionary Biology and Ecology
Generative AI can also contribute to understanding evolution and biodiversity by:
- Simulating genetic evolution over time.
- Predicting species adaptation to climate change.
- Modeling ecosystem interactions to study biodiversity.
Future Potential:
- AI-driven genetic simulations could unravel evolutionary mysteries.
- Conservation efforts could be enhanced through AI-generated species data.
- AI models could predict the impact of climate change on biological systems.
Impact of Generative AI on Bioinformatics Innovation
Generative AI (GenAI) is poised to have a profound impact on bioinformatics innovation, transforming research and healthcare through machine learning models in ways that were previously unimaginable. By harnessing the power of AI to generate novel insights, predict biological outcomes, and optimize processes, GenAI is helping to address long-standing challenges and accelerating the pace of discovery. Here’s a look at the key ways of Gen AI and the future of bioinformatics:
- Accelerating Drug Discovery and Development
- Precision Medicine and Personalized Treatment
- Enhancing Disease Prediction and Diagnostics
- Optimizing Gene Editing and Synthetic Biology
- Automating Research and Data Analysis
- Improving Clinical Decision Support Systems
- Fostering Interdisciplinary Collaboration
- Enhancing Biotechnology and Industrial Applications
- Improving Environmental and Agricultural Biotechnology
- Ethical and Regulatory Advances
Challenges and Ethical Considerations
Despite its immense potential, integrating GenAI into bioinformatics comes with challenges:
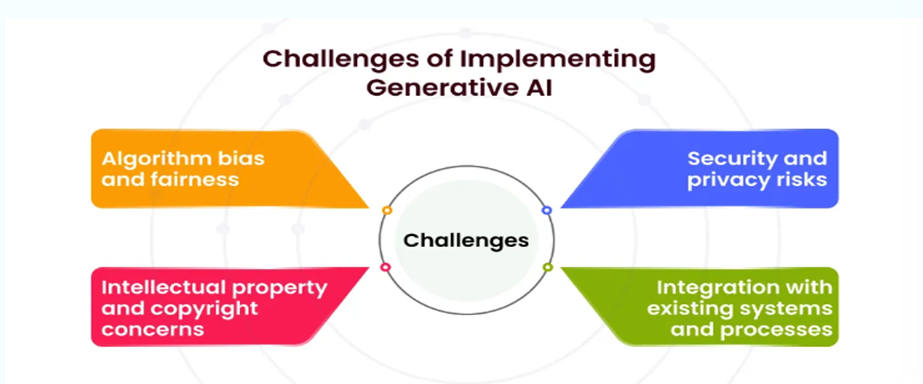
1. Data Privacy and Security
- Ethical concerns regarding AI-generated genomic data.
- Risk of misuse of synthetic biological sequences.
- Ensuring secure storage and processing of patient data.
2. Reliability and Validation
- AI-generated data must be rigorously validated against real-world observations.
- Lack of interpretability in AI models raises concerns in clinical decision-making.
- Generative AI models can sometimes hallucinate false patterns, leading to misleading conclusions.
3. Regulatory and Legal Frameworks
- Need for regulatory guidelines for AI-driven drug design.
- Addressing intellectual property rights in AI-generated biomolecules.
- Ensuring AI-generated recommendations align with medical standards.
4. Computational and Resource Constraints
- Training GenAI models requires high computational power.
- Storage and processing of large-scale biological data require advanced infrastructure.
- Accessibility of AI tools in low-resource settings is a concern.
The Road Ahead: What’s Next for Gen AI in Bioinformatics?
As we look into the future, the scope of Generative AI and the future of bioinformatics will continue to expand with:
- Integration with Quantum Computing: Speeding up molecular simulations and genomic analyses.
- AI-Driven Bio-Manufacturing: Designing synthetic cells and organisms for industrial applications.
- Next-Generation AI Models: More interpretable and explainable AI for healthcare applications.
- Automated Scientific Discovery: AI formulating hypotheses and conducting experiments autonomously.
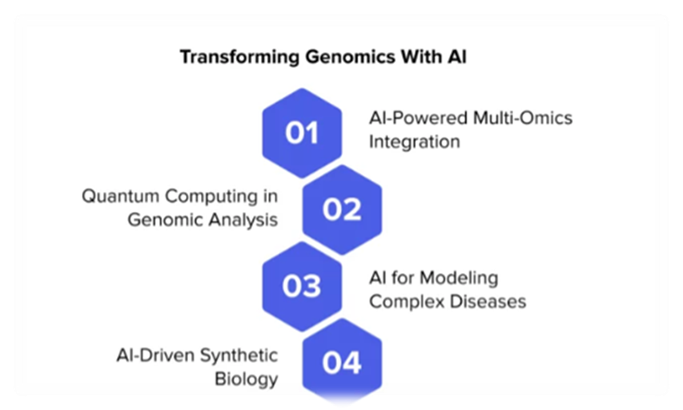
CONCLUSION
Generative AI and the future in bioinformatics is incredibly promising, with the potential to accelerate scientific discoveries and improve healthcare outcomes. From drug discovery to personalized medicine, machine learning models will continue to reshape the field of bioinformatics, making biological research more efficient, precise, and accessible. As technology advances, interdisciplinary collaboration between AI specialists, biologists, and healthcare professionals will be essential to unlock the full potential of AI models in bioinformatics.